MIT and MGH develop Sybil, a deep learning model for lung cancer risk assessment
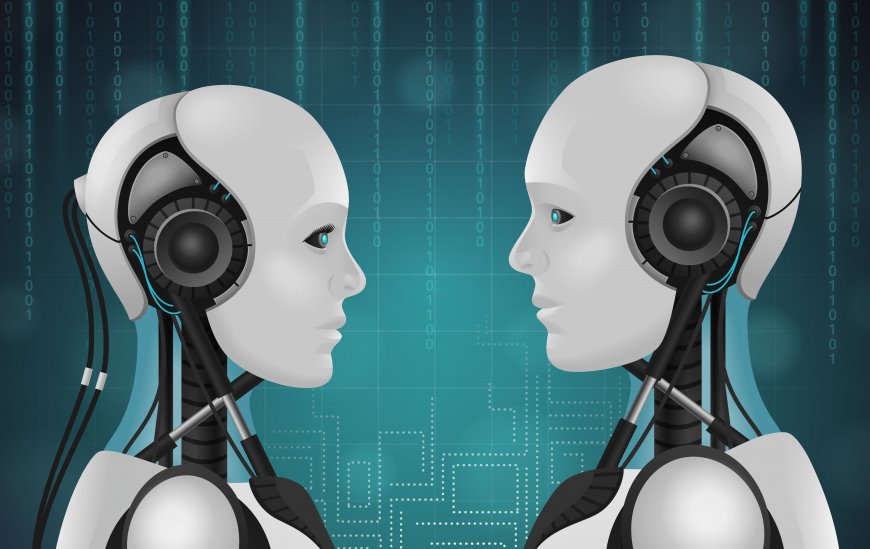
Lung cancer is a terrible disease. According to the World Health Organization, lung cancer has become one of the most common causes of death worldwide, causing nearly 2.21 million deaths in 2020 alone. What's more, the disease is progressive - in the majority of patients, lung cancer starts with mild, unrecognizable symptoms, but rapidly evolves into a serious, life-threatening condition. Fortunately, the treatment of lung cancer patients has evolved dramatically over the past two decades. But for now, early detection remains the only way to significantly reduce lung cancer mortality.
Recently, the Massachusetts Institute of Technology (MIT) and Massachusetts General Hospital (MGH) announced the development of a deep learning model called Sybil that can predict lung cancer risk using a single CT scan. The study, which was published last week in the Journal of Clinical Oncology, discusses the possibility of "serving high-risk populations with a personalized tool for assessing potential cancer risk". The study leaders hypothesized that "a set of deep-learning individual risk prediction models evaluating full-volume LDCT (low-dose computed tomography) data could be built to provide reliable conclusions without additional demographic or clinical data."
The model follows a very simple rationale: "LDCT images contain information that can be used to predict future lung cancer risk with higher accuracy than existing identifiable features (e.g., lung nodules)." To this end, the developers sought to "develop and validate a deep learning algorithm to predict the risk of lung cancer over the next six years from a single LDCT scan and assess its potential clinical impact."
Overall, the study is now a milestone success: Sybil was able to predict patients' lung cancer risk with some degree of accuracy, relying only on data from a single LDCT.
However, it must be recognized that the emergence of the algorithm is highly symbolic and a potential game-changing force in diagnosis and treatment.
Such a powerful diagnostic method could be beyond what was previously thought possible. The ability of a tool to predict long-term disease trends on the basis of a single CT scan alone promises to address numerous real-world problems, particularly the implementation of early treatment and the reduction of patient mortality.
There may be concerns that such systems are trying to take away doctors' jobs, emphasizing that no AI system has yet to replace human doctors in terms of judgment and clinical care. That's right, but such systems are not intended to replace doctors, but to provide them with efficient and reliable job aids.
Systems like Sybil can be used as a recommendation tool, flagging features that may be indicative of a medical condition to the physician, who will then make a choice of suggestions based on his or her clinical experience. This has the potential to not only improve the efficiency of consultations, but also improve diagnostic accuracy by assisting in the "checking" process.
Although there is still a lot of work to be done. Scientists, developers, and innovators can't stop exploring not only the specific algorithms and systems themselves, but also the nuances of bringing this technology into the clinic. If a safe, ethical, and effective implementation route can be found, this technology could have a huge impact on improving patient care and ultimately disrupt the entire treatment system as we know it.
What's Your Reaction?
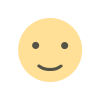
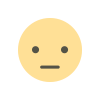
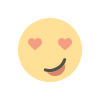
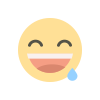
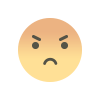
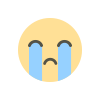
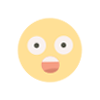